We introduce you to data analysis and provide a seven-step guide for how to analyse customer data and meet business objectives.
What Is Customer Data Analysis?
Data analysis is the process of mining raw data for insights. These insights will be relevant to your organization’s key goals.
For example, your company might decide to run customer data analysis to:
- Understand customer demand
- Formulate metric scores
- Run root cause analysis
- Discover how people judge your customer experience
- Assess areas of high customer effort
These are just some of the reasons why a business may want to run data analysis of either quantitative or qualitative data – or perhaps even both.
What’s the difference between quantitative or qualitative data, you ask? Well:
Quantitative Data – This data is expressed through numbers that can be counted and measured. It is often presented visually in tables and graphs. It can therefore be useful to analyse this data to inform your decision-making, but it will not usually enlighten you as to how you can solve a problem.
Qualitative Data – This data is illustrative and can be categorized in order to define a problem. It is also often used to add context to quantitative findings, as qualitative data is non-numeric. A customer’s personal information (gender, address, etc.) is an example of qualitative data.
The data sets, be they quantitative or qualitative, that you mine will depend very much on what business insights you are looking to obtain.
How to Analyse Customer Data
We have put together a list of seven steps to analyse customer data effectively:
1. Decide on Your Objectives
So, this is the first step in any customer data analysis, deciding on your objectives.
Before you start gathering your data, talk to your stakeholders and decide on your key strategic goal.
Also, talk to stakeholders to gain a clear understanding of the types of insights they are looking for and how you can use those to meet the objective.
Then, it’s really important that the team who will be running the data analysis all have a shared understanding of that goal.
If everyone has different definitions for your objective, everybody will go off in different directions. That’s when things get expensive, difficult and frustrating. There needs to be coherence in your process.
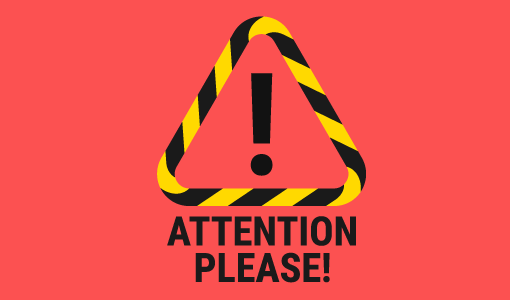
Warning. Before you start looking through data, it’s also important that you don’t hold any preconceived narratives about how you can change in order to meet your objective.
Warning. Before you start looking through data, it’s also important that you don’t hold any preconceived narratives about how you can change in order to meet your objective. If you have these, you will only use the data to look to confirm your own ideas.
The key here is to avoid rebadging storytelling as analysis. If you do this, you will believe your own stories and move on from there. This is something that happens in politics, much to its detriment.
To help remove these narratives, it’s good to have a data analysis team that includes people from outside of the contact centre. Narrative doesn’t last long when it is being looked at from multiple perspectives.
2. Identify Business Levers / Frameworks
Too often companies approach customer data analysis by saying: “Right, I have this data and these analytics tools, what can they give me and what can I therefore do with this data?”
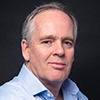
Duncan White
“This approach is completely the wrong way around,” says Duncan White, Managing Director at horizon2.
“Data analysis starts with clearly defining the objective and then considering how can you use your current data supplies and business levers to achieve that.”
The most critical business lever to consider is your customer journey framework. Look at your customer touchpoints. Think about where you can collect the most meaningful data that will help you meet your objectives.
When you think about this, you also want to consider the tools that you have for data collection and how you can use them in a way that doesn’t disrupt customer experience. These tools may include:
- Speech / Contact centre analytics
- Call centre reporting
- Voice of the Customer (VoC) tools
- Journey tracking tools
- Website analytics, cookies and IP addresses
- Transactional insights
If you want stakeholders to invest so you can obtain more tools for customer data analysis, really look at the biggest positive impact of the objective. There may be lots of associated benefits to achieving that objective that are worth highlighting in the hope of attaining more budget.
For more ideas on how to build a business case for improved data analysis tools, visit: 11 Ways to Secure More Budget for Your Contact Centre
3. Collect Your Data
Have the right biomass of information and eventually a diamond will appear.
When trying to refine your data to make improvements, you’ve got to start out with the right raw material. Have the right biomass of information and eventually a diamond will appear.
In the last section we discussed some of the tools that you can use for data collection and why it is good to look at your customer journey framework to spot the right place to collect data. But there is a lot more to data collection than that.
For example, you also need to think about:
- How are we going to store and organize the data that we collect?
- What will we do to protect that data and stay compliant?
- When we are collecting the data, how transparent should we be with customers?
All of these security considerations are key, as is how you organize the data so it is easy to analyse when the time comes. But let’s also consider customer experience.
Think about: how can we collect the right data at the right time, in an effortless way?
From your journey framework, you know where certain types of customers are likely to go, so think about how you can source customer intelligence in a non-invasive, compliant way. That is the heart of collecting data.
4. Clean Your Data
Now you have collected, stored and organized your data, it’s time to clean it. This will mean that the insights that you take from your customer data analysis will be both accurate and reliable.
There are four basic stages in cleaning data. These include:
- Step 1 – Inspecting the data to look for errors and inconsistencies.
- Step 2 – Fixing or removing those outliers.
- Step 3 – Testing your changes to verify their validity.
- Step 4 – Logging those changes and reporting on overall data quality.
This is a great process to follow for cleaning data. But to help you out a little more, take a look at some of the suggestions below for improving you cleaning process and therefore the accuracy of your insights.
Follow a Checklist – Checklists ensure that you have taken all of the necessary steps in the data cleansing process and, by using them, you can guarantee that everybody follows the exact same process when they are cleaning data. This helps to guard against conflicting results.
Question Your Outliers – Look for patterns in your outliers, to ensure that what you think are outliers are not just indicators of a new business as usual. If you are fixing your outliers and adding them back in, remember that you can’t just set the value of that data as zero – maybe you need to use a deviation of the figure? It depends on what you want to do with the data next.
If you are fixing your outliers and adding them back in, remember that you can’t just set the value of that data as zero – maybe you need to use a deviation of the figure?
Correct the Additional Source – If you are going to clean data, go back to the original source and clean it for everybody. It makes no sense for everybody to have to clean it themselves before they can start digging for insights.
Make a Record – Cleansing data means making a number of decisions one after another, when questioning outlier-after-outlier. Remembering all of those possible changes is going to be an impossible task. But making a record will enable you to answer questions when revisiting previous decisions. It will also allow you to replicate the same data-cleansing process in the future.
Talk to the Team – When you clean data, you will often take on the detective role. But before you make a judgement call, run it past your team first. They need to know how you have processed the data should they then get involved in modelling the data and reporting on it.
For more insights on this topic, read our article: A Step-by-Step Guide to a Customer Data Cleansing Process
5. Model the Data
Data modelling is the method of designing a process that filters the data that you have just cleaned.
A data model segments your data and pulls it in different directions, based on rules that you input into your model – e.g. compliance regulations – in order to extract insights.
Sounds complicated, right? Well, a good starting point for data modelling is to create an entity relationship diagram (ERD). This is explained a little more in the video below.
Once you have done this, you can much more easily manage, update and retrieve your data, so that it becomes simpler to analyse.
This means that you can move onto analysing your data, which you can perhaps do by using the following techniques:
- Cluster Analysis – This technique involves comparing two sets of data on a graph and assessing their relationship with one another by looking for clusters.
- Cohort Analysis – Cohort analysis involves dividing data into cohorts and assessing how their behaviour changes over time.
- Predictive Analysis – This technique looks at data that represents patterns in customer behaviour, so you can start predicting what they will do next.
- Regression Analysis – Regression analysis is a technique where you analyse two sets of data and look for correlation between the two.
While each of these data analysis techniques may prove useful, data modelling will also enable you to run each of the six types of call centre data analysis highlighted after this seven-step process.
It must be said, though, that an ERD isn’t the only data modelling technique that you can use. If you wish to look into other methods, you can also research “unified modelling language” (UML) and “data dictionary”.
6. Use Customer Data to Convince Stakeholders
You’ve now modelled your data and applied some of the data analysis techniques highlighted above and have developed data-driven insights that meet your initial objectives.
But when you are dealing with strategic challenges within an organization, the next stage will mean that you will likely have to bring multiple teams and multiple departments together.
Within these teams and departments, you will likely uncover conflicts of interest – but if you’ve done your analysis properly and your findings are accurately described, that helps. Evidence is your key to avoiding conflict.
Getting that solid evidence together without having some sort of “paralysis by analysis” is the art.
Getting that solid evidence together without having some sort of “paralysis by analysis” is the art. There needs to be an efficiency in it, with a clear process that everyone is aware of, so nobody questions the key lessons learnt in your debrief.
You need to show teams the consequences of their actions in a safe environment, where nobody gets defensive and makes assumptions that just because they’ve been doing something for a long time, that means it must be the best thing to do. Often it’s not.
People need to see your data analysis team as the analytic engine that sits at the heart of your business to turbo-charge the potential that already exists within your organization.
7. Optimize and Repeat
At the end of every data analysis journey, it’s good to question what you could have done differently, in order to optimize the process in the future.
Maybe you can look into new tools to collect data, better ways to clean data and maybe even play with better data visualization techniques – in order to better spot insights and make a point to the wider team in your debriefs.
Play with better data visualization techniques – in order to better spot insights and make a point to the wider team in your debriefs.
Then repeat the process, not only to see whether the data-driven changes made have enabled you to reach your initial objective, but also with a new objective.
Obviously, a new objective will affect the data that you collect and the analysis techniques that you use, but if you can really get to grips with this seven-step process – and get buy-in from the wider organization – you can use data to back up some really great decision-making.
6 Types of Data Analysis to Use in the Call Centre
There is no greater source of data and insight within an organization than the contact centre.
With this in mind, Jonathan Wax of NICE Nexidia introduces us to six great ways to analyse contact centre data, in order to provide value to the entire business.
- Interaction Analytics – By analysing customer interactions, you can uncover context, sentiment and behaviour, as well as intent. This enables you to run root cause analysis and predictive modelling, find event triggers and report on key metrics.
- Desktop Analytics – By using desktop analytics, you can map and monitor service processes. This enables you to measure skill vs. will, find the true process cycle time and calculate “first touch resolution”.
- Journey Analytics – Journey analytics provides you with a multichannel picture of your individual and overall customer journeys, helping you to understand why customers switch between certain channels and behave how they do.
- IVR Optimization – Data that is stored within your IVR can be used to identify “choke points” or bottlenecks to increase overall containment.
- Voice of the Customer (VoC) Analytics – VoC analytics includes the analysis of both direct and indirect feedback. A specialist VoC tool can provide teams with role-specific charts, reports and alerts, helping to deliver business-critical insights.
- Predictive Behavioural Analytics – Using call routing software, you can match customers with agents by pairing them up based on their personality style and other information – e.g. age, address and interaction history – in order to improve rapport building.
For ideas for how you can analyse customer data, read our article: 9 Ways to Analyse Customer Data
The Key Message – Take Your Customer Data Analysis Beyond the Call Centre
The trouble with most customer data analysis projects is that they often don’t extend beyond a single department, and most customer data analysis is done in the call centre.
This was understandable in the days before customer journey mapping and the strong focus on the overall customer experience.
Yet nowadays, contact centre advisors are generally very good, well coached and knowledgeable, and the problems that people have are more to do with the process, the outcome and their journeys.
On top of this, Duncan White adds: “The problem with just looking at the contact centre is that you can improve your piece but neglect something that’s either downstream or upstream that is the key thing to change and will have a much bigger impact on customer satisfaction and sentiment.”
So, organizations are tending to do more analysis on the overall customer journey and not individual touchpoints. This is great, but difficult to achieve.
The aim should be to have a data analysis team at the heart of your business, which every department buys into. It should provide clear data-driven insights on how everyone can pull together to meet your primary organization objectives.
Good luck!
For more insights into how to analyse data, read our articles:
- 16 of the Best Uses for… Contact Centre Analytics
- Keeping Your Call Centre Data Safe: What You Need to Know
- How to Reduce Agent Time Spent on Data Entry
Author: Robyn Coppell
Published On: 23rd Nov 2020 - Last modified: 12th Nov 2024
Read more about - Technology, Analytics, Measurement, NICE