We present the latest insight into customer service artificial intelligence (AI) and how it can be implemented in contact centres.
AI Reality vs. AI Hype
Don’t get carried away with the hype. The whole implementation and deployment of AI technologies in the real world remains a big challenge, and that process is something that executives in businesses today are starting to realize.
Consider the following statistics from a 2019 Deloitte report: in 2017, 76% of executives believed that AI could substantially transform their business within three years’ time. This fell to 56% in 2018.
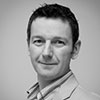
David Naylor
While this may seem to be all “doom and gloom”, some would actually consider this situation to be great, because it shows that organizations are putting more thought into AI, as David Naylor, Founder of Humanotics, believes.
“Executives now understand that there many different components and applications of AI, allowing them to think more about building it into their roadmap of continuous improvement and considering, more deeply, where is best to use it,” says David.
The bottom line is, build your roadmap, learn how the technology works and go from there.
AI Generates Natural Language… But Not Yet Conversations
AI technology has advanced much quicker in some areas as opposed to others. Language understanding is one area that has shown much promise within the customer service space.
In fact, according to the General Language Understanding Evaluation (GLUE) benchmark, since the beginning of January 2019, machine performance in understanding natural language has actually exceeded the human benchmark.
The results are based on comprehension tests – similar to what you would have in a school exam – and show very good promise. But understanding language is just one aspect of how you build conversations.
Understanding language is just one aspect of how you build conversations.
Where AI isn’t so good is understanding the context of the whole conversation and generating natural language responses that deal with situations where people deviate from the “script”. The AI software that’s available at the moment is still very simplistic in this respect.
So what can we use AI Natural Language Understanding (NLU) for, at this time?
In the wider world, there are two interesting ways that we have recently seen:
- Filling gaps in written communication with what was likely intended to be said.
- Taking natural language and creating new content (this can be used to create fake news!)
While these “uses” are promising, it is a one-sided thing. Taking text to generate some more text is very different from creating dynamic conversations between humans and machines.
So, as a lot of organizations that use chatbots for customer service have come to realize, conversations with robots are still created in a very scripted way.
Things are advancing. For example, Google recently released a new version of its chatbot conversation toolkit called Dialogflow. This now remembers precisely the point you’ve reached in the flow – the conversation ‘state’ – and so it is easier for the designer to control what the bot should be able to respond to next.
However, in terms of using AI that can manage this process itself so you can avoid scripting each stage and so fully automate customer conversations, there is still a lot more work to be done.
Mapping AI Into the Customer Contact Strategy
The key message here is that AI isn’t this wonderful panacea of out-of-the-box solutions to all of your contact centre problems – which it was perhaps sold to everyone as a few years ago.
A key principle to adding AI into your customer service mix is to first consider the key interactions that you have with customers. Then you can look into where AI can be added to help drive improvements – whether that’s in customer satisfaction, growing revenue or other key business outcomes.
A good framework to help you do this is the Humanotics Value-Irritant Model, as it enables you to structure your thinking in terms of the types of interactions you are having and where AI can add value. The model is laid out below.
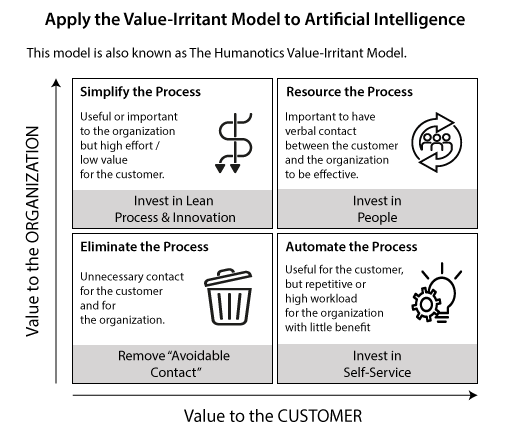
Consider your most common contact reasons one at a time. For every reason, consider:
- How valuable is that contact to your customers?
- How valuable is that contact to your organization?
As you can see from the chart above, if the contact is not valuable to both, we want to eliminate that contact. It is failure demand, so we need to work on our processes to eliminate that contact reason altogether.
If it’s of value to the customer, but an irritant to the organization, then think about how you can automate that process. How can you give the customer what they want but avoid cost and human interaction as a result of fulfilling the customer’s need?
If it’s of value to the organization, but an irritant to the customer, then think about how you can simplify that, so that customers can go through these processes (e.g. compliance) in a matter of minutes, rather than potentially hours or even days.
If it’s of value to both you need to resource it and this means giving your people the tools and the skills to support customers. For instance, if a customer can’t pay their bill, how can you deal with that in a very human way?
There are lots of ways for you to eliminate, automate, simplify and resource contact reasons and, to do each of these jobs, there are AI applications that might be able to help you.
The AI applications that are included in the chart below – as put forward by David – are a great example of how you can do this:
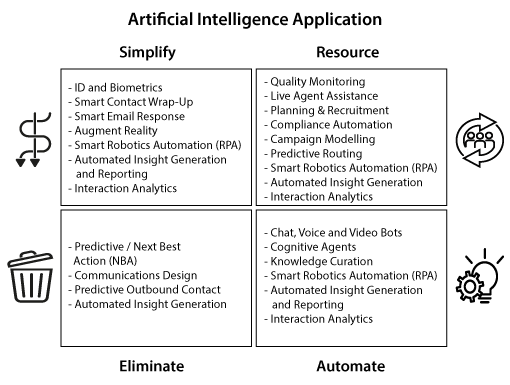
This is a very helpful guide, but when you start to plan out how and where you will use these AI applications, you need to also be thinking about what your business objectives actually are…
Why Are Organizations Implementing Customer Service AI?
We asked our readers to highlight their biggest drivers for implementing AI, and the results are as follows:
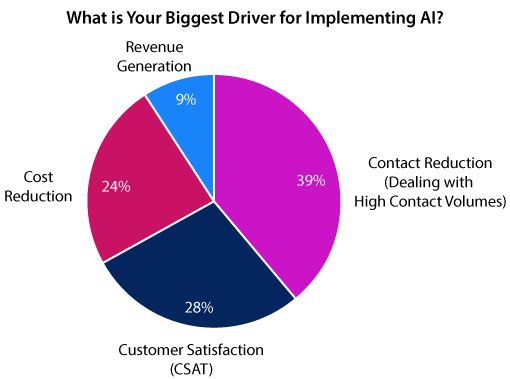
The data included in this chart is sourced from our webinar: AI Trends to Keep an Eye On
It is interesting to see that contact reduction came out on top here and, if this is your number-one priority, go back to the value-irritant grid and focus first on the “eliminate” and “automate” sections – as these are most relevant to your primary objective.
If your biggest driver is customer satisfaction, you want to focus on the right-hand side of the grid (i.e. the areas that signify value to the customer) and focus first on the “resource” and “automate” sections.
Whatever your business goal, having a framework to map out AI into your customer service strategy is key. This may just be the value-irritant model, but there are other key frameworks, like a customer journey map.
For an introduction to creating a customer journey map framework, read our article: 5 Steps to Creating a Customer Journey Map
5 Exciting Customer Service AI Use Cases
Here are five interesting use cases of customer service AI innovations.
1. Real-Time Speech Analytics
Speech analytics (and speech recognition) is by far the biggest use case of AI in customer service. It is the technology that converts audio conversations into text, using a combination of linguistic rules and artificial neural networks.
While these systems were quite niche in 2010, they are always improving and they have gained more popularity over the past decade.
Some of these use AI to present key findings in a dashboard to advisors and team leaders.
Why? Well, for starters there is lots of information available to you within real-time speech analytics systems. Some of these use AI to present key findings in a dashboard to advisors and team leaders.
The problem with customer service dashboards is that they too often provide “information overload”, where instead of just showing customer service reps key learnings from each call, they monitor every statistic possible – so the rep has no idea where to look.
Customizing the agent desktop to include insights from AI-based real-time speech analytics is therefore a promising use of AI in contact centres, as it can be programmed on certain contact types to guide the advisor. This could be pushing articles from a knowledge base based on a question the customer has asked, telling the advisor to slow down or even to use more positive language.
Another good use of real-time speech analytics is in “proactive pre-complaint resolution”.
This involves your analytics systems learning over time to recognize patterns in customer behaviour and predicting that they will soon make a complaint.
Armed with this insight, the contact centre can then give these customers an outbound call, to improve customer retention rates and satisfaction.
There are lots more uses for speech analytics than just these, though. To find out more, check out our article: 16 of the Best Uses for… Contact Centre Analytics
2. Conversational Bots
Over the past few years, many organizations have implemented very simple bots, some of which contain very little AI. These have enjoyed mixed success.
While FAQ bots are now common, they are not very sophisticated and respond to individual questions with answers pulled from content on webpages.
The ability of AI is limited to the understanding of each ‘utterance’ made by a customer rather than the whole conversation. Even then, a bot would not be able to distinguish between “I want to cancel my insurance” and “You’ve cancelled my insurance.”
Bots that allow the customer to complete a task such as chase up an order status, for example, are appearing, but they rely on customers sticking to the script.
Tools are developing quickly that allow bots to remember the history of a conversation, but perhaps only the last 2 or 3 ‘exchanges’, and these are still heavily script-driven, which means they can get very complex.
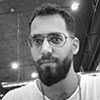
Noam Mor
Yet, according to Noam Mor, an AI Conversation Design Team Lead at Vonage, this is not enough.
“An AI Virtual Assistant should understand the context of a conversation, through information that is collected in the early stages of a call, and then reuse it later on, if necessary,” says Noam.
So, where is all of this “bot-talk” leading us? David Naylor believes that we are heading into a world of “cognitive agents” – instead of individual human agents and bots, as he explains in the video below.
The idea of bringing AI tools into conversations and using them to adapt the conversation – instead of just using them offline to analyse what happened – is potentially a powerful approach for the future contact centre.
For some, this is the future of conversational bots over the next couple of decades, in generating insights that allow customer service reps to have better conversations.
To uncover some interesting misconceptions around conversational bots, read our article: 7 Things They Won’t Tell You About Installing a Chatbot
3. Video Emotional Insights
Most customers now contact you through their smartphones. These are increasingly clever devices that can give us lots more insights into our customers, if they are willing to share those with us.
For example, smartphones come with cameras. If the customer gives us permission to take over their camera, we can use AI tools to generate lots of interesting video emotional insights.
If the customer gives us permission to take over their camera, we can use AI tools to generate lots of interesting video emotional insights.
With access to the camera, we can pick up data relating to their facial expressions and predict their mood from the movement of their eyebrows, lips and so on.
Yet this information, by itself, is just information. The challenge is how you take this extra data and embed it into behavioural models that will help you to make decisions around how you change the course of the conversation with the customer as a result.
While this technology is out there now and you could go to imotions.com to implement this AI tool to start tracking emotional insights – it’s how you use these insights to improve your customer and business outcomes which is the tricky bit.
4. Machine Learning Predictive Models
There are a number of machine learning models that are designed to predict customer behaviour, and these are starting to be implemented within sales teams to target certain customers and improve sales.
Raiffeisen Bank used a predictive model to identify seven sales phrases that are used in successful loan sales calls.
In terms of a real example, Raiffeisen Bank used a predictive model to identify seven sales phrases that are used in successful loan sales calls, which helped to produce a great uplift in sales performance.
One of those successful phrases was: “I can immediately activate the credit card for you.”
The bank blind-tested these phrases with two teams – using an A/B testing methodology over two weeks – which found great value in machine learning for sales.
There are other machine learning models that can be used to predict customer behaviour – with the most common being in predicting customer churn – but they do take a long time to perfect.
5. Journey Simplification
Smart robotic process automation (RPA) can be used to simplify paper-based processes, and this AI tool can really help to reduce effort within customer journeys.
Take a look at this “old-school” customer and company journey for completing a mortgage application.
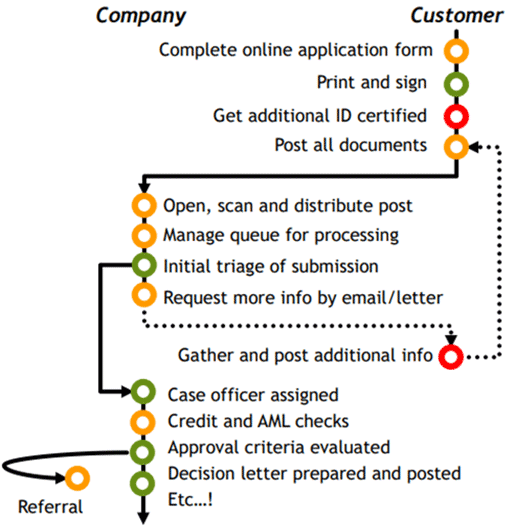
This journey, which would have taken days or maybe even weeks, can be automated and turned into 15-minute process, via a smartphone.
Just take a look at the new journey, which was made possible by Humanotics working with Nivo as well as other AI-based tools, including a secure chatbot, machine learning and biometrics.
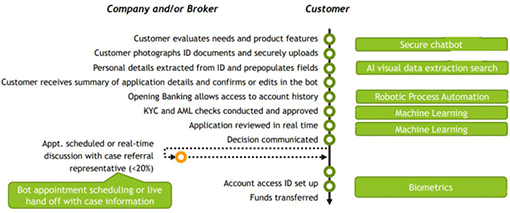
In this example, we can see how smart RPA, with some cleverly placed AI tools built around it, can help to redesign and simplify customer journeys.
To find out more use cases for AI within the contact centre, read our article: 12 Top Uses of Artificial Intelligence in the Contact Centre
Is the Future of Customer Service in Hyper-Augmented Reality?
When looking into the future of customer service, you should not be dictated to by AI tool vendors as to how you support customers as a business.
The video below shows a hyper-augmented reality, where many AI technologies are being used all at once in a crazy example of hyper-augmented reality.
With the metaverse, customers will be able to take a 3D tour and do research before committing to an item or location using VR platforms and hardware.
This can be quite an unsettling watch. But, when viewing the video, it’s important to recognize that the technology is not too far away and AI is a tool that has the potential to take our world in a number of different directions – not all great. It’s therefore good to ask yourself: what future do we want to create?
“As an industry, I think that we need to control this. We need to deliver what we want as a society, as a business and for our customers and using the best bits of these AI components,” concludes David Naylor.
Key Takeaways for Implementing Customer Service AI
We have brought you several perspectives on the future of customer service AI within this article. So let’s finish with some key takeaways:
- Our goal needs to be balancing the use of technology with better people, skills and process improvement.
- Start by building your roadmap, then learn how the technology works and go from there.
- Use a framework like the value-irritant grid to add AI where it has the most value, in reference to your key organizational goals.
We hope that this summary of customer service AI has been helpful, and good luck in creating an AI strategy for your organization!
To find even more guidance on how to use AI within your customer service operation, read our articles:
- 14 New Uses of Artificial Intelligence in Customer Service
- Artificial Intelligence in the Contact Centre: What You Should REALLY Know
- The 10 Things They Won’t Tell You About Artificial Intelligence
For more from David Naylor on the use of AI in the contact centre and customer service, listen to the following episode of The Contact Centre Podcast.
The Contact Centre Podcast – Episode 4:
Contact Centre Ai: What Are Your Options?
For more information on this podcast visit Podcast: Contact Centre AI: What are your options?
Author: Robyn Coppell
Published On: 1st Feb 2021 - Last modified: 11th Nov 2022
Read more about - Technology, Artificial Intelligence, Chatbots, Customer Service, Vonage