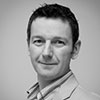
The Contact Centre Podcast: Episode Four
In this episode, Dr. David Naylor of Humanotics discusses how contact centres are using different forms of artificial intelligence (AI), while dissecting some of the hype surrounding the technology.
As part of our discussion, we remove many AI miscoceptions while discussing the promise of technologies such as predictive routing, biometrics and chatbots.
To listen to the podcast directly from this webpage, just hit the play button below:
The Contact Centre Podcast – Episode 4:
Contact Centre Ai: What Are Your Options?
This podcast was made possible by our sponsor, Genesys. We now have a new link to visit their website, instead of the link mentioned in the podcast to request a demo.
So, to find out more about Genesys, simply visit their website
Podcast Time Stamps
- 1:55 – Uncovering the AI Hype
- 5:11 – Gathering the Right Data
- 7:47 – Predicting Customer Behaviour
- 13:06 – Using Predictive Routing
- 19:30 – Chatbot Misconceptions
- 22:44 – The Best Applications of Chatbots
- 27:18 – Implementing Robotic Process Automation
Here is a Transcript to the Podcast
Charlie Mitchell: I remember hearing a presentation that you gave and you said that AI in the contact centre was a topic that was discussed all the way back in the 1990s, but then it went away for a little while until recently, and now all of a sudden there’s a lot of hype around the technology. Why do you think this is?
David Naylor: I think, go back to the early 1990s when I did my PhD in AI, I didn’t think I’d be talking about it 30 years on. It is quite nice to be able to do that. What was happening in those days was very much around using fairly slow processing power, limited amounts of data. It was very much early days of trying to make some of these machine-learning models, which I think is what’s caught the imagination in the last few years. Models where you train the AI with data to represent, with inputs and outputs that represent a certain scenario, you’re trying to model and replicate. In those early days we were limited with what we could achieve in terms of scaling those things up to real-world applications. What’s happened in the last sort of five, 10 years is because of the growth of data, a lot of which has come off mobile phones, so consumer usage of phones has created tons and tons of data to the likes of Google.
That means that they can start to use that to analyse customers and analyse behaviour and from there predict things, whether it’s for sales or whether it’s for service, and can then deliver those services through the phone, as well as the processing power. So we’ve got the architectures that Google’s built in the cloud that have taken their technology and their solutions that are developed internally and deployed those to the wider world now, so that anyone can take these same models and build their own machine learning, artificial intelligence models they can use in their own business. So we’ve got the processing power, we’ve got lots of data, we’ve got the… there’s the frameworks, if you like, is probably the best way to describe them, that people can use for a very low cost start point. It’s just sign up, get an account and you can start building an artificial intelligence-based model.
So it’s really transformed the whole artificial intelligence world. It’s totally different to what we were doing 30 years ago. And it’s great to see some of the people trying things. I think the danger is that there’s a lot of hype that’s been attached to this in terms of it being able to solve lots of problems. The challenge is ensuring that you’re applying it to the right problems because not every problem is one that will get the most out of these models. And I think at the moment a lot of companies are just talking about AI and saying, “we’ve got AI built into our product.” Actually it probably had some analytics and some logic that is doing the same job as the AI is doing for the last 10 years or more, and just what the machine-learning models can now do is probably do it slightly better, but not necessarily significantly better.
So we need to be careful that when we’re looking at these claims around AI today, that there truly is some benefits from what that AI is proposing to do.
Charlie: I think you’ve raised a very interesting point now that the difference between what is mediocre AI and what is great AI is just the amount of data that you have access to. Do you have any tips for obtaining data that’s stored beyond the contact centre?
David: Well, I think that the important thing about having access to data in an organization is that everyone needs to understand the value of that data and be willing to share it across the business. And I think we still have a lot of data silo mentality across our organizations where marketing hangs onto its data, customer service hangs onto its data, finance, et cetera.
It’s all seen as belonging to that team, for instance. But I think the challenge is saying, right, if we have a proper strategy around AI, that means that we need to have a proper strategy around our data. And therefore organizations that are leading in this space have put in place that data information strategy to support the development of use of data with AI. And just not just with AI, but just in general, sort of generally using that data better across the business. So break down those silos, whether that’s physically making sure that data gets centralized through data lakes or whatever is appropriate for the organization. But making sure that there’s a physical place where you can access that data. And also then the policies and procedures in place that allow you to use that data. And I think there’s clearly, there’s a lot of concern about how data does get used, naturally important today with GDPR and all those pay data protection regulations.
So as well as having that physical side in place, it’s also important to have the processes in place to support that. So it sounds like it’s a big task. It doesn’t have to be a big task. I think there’s two ways to think about this, as the underlying architecture that you need to process and policies to support that access. But then it’s important to have experimentation going on. So you need to understand what data is useful. So you need to be trying things and experimenting with data to see how it can be useful to the organization. And those things then provide a business case that describes and explains why you should move towards a more centralized approach to your data. So often the business case to put data in one place is actually really hard to justify. Most organizations just don’t see the value. So, do some experimentation work between departments where you’ve got some like-minded individuals and common goals. Prove there’s some value in bringing that data together, investing in more centralized repositories and approaches, and then from there, it should help to create that common view of sharing and benefiting from data.
Charlie: Excellent. I think there’s lots to think about as you’ve gone through there in obtaining that data. But once we have it, I think one of the best use cases of AI I’ve heard is in predicting customer behaviours. You can use that to then retain customers. So could you tell us a little bit about how this might work?
David: Sure. I mean, so there’s two sides to AI applications as far as I see it within customer management space. So there’s the interaction side, which I’m sure we’ll talk about shortly, and how you use data in real-time interactions. And then there’s the prediction side, whether that’s to do with predicting next activities in terms of, will this customer buy a product if we offer it to them, or what’s the likelihood of this customer churning? So those sort of models are things which take time to develop, and we have to work out what are the factors that are important and driving that behaviour. And we’ve got probably got quite a lot of experience in lots of businesses of putting together simple models that say if a customer called in the last month and has complained and has called again and the complaint’s not resolved and they’re outside of their contract, the chances are they’re going to want to cancel.
David: So those simple models are okay and you can keep using those and you know that’s going to give you a certain level of success in terms of churn management. What AI allows you to do is actually go beyond really what you can see in that simple data and go almost a bit further back in time and start putting together more information about what the customer’s purchased in the past and how many times they do make contact with you. And what’s the sort of issues they’ve had when they’ve made contact with you. You don’t necessarily need to come up with a defined premise that says if they do this and if they do that, they’re going to churn. The data will tell you that, if you select the right input data and then look at what’s the profile of somebody who’s churned, what’s the profile of customers who don’t churn and build the model so that it learns both types of customer.
You’ve got to be careful that you don’t just throw everything in. You know, it’s still important to think about what are the potential bits of data that could affect it, but you don’t need to really understand too precisely what does affect it. The model will help you to get to that point. So those are some really good examples of what people are doing now. And there are other things like fraud. Fraud is a good example of being able to manage fraud better. That’s something that a certain number of clients in the financial services space are really sort of making headway with in terms of sort of using AI.
Charlie: Yeah, I think that’s very interesting that you bring up fraud because that’s definitely a topic I’ve heard AI now being used a lot in that area. Do you envisage more and more organizations turning to AI to help improve their security?
David: Definitely, definitely. I was talking to one insurance company in the last few weeks and with traditional models they managed to go a fair way towards reducing fraud. They learned a lot about what drives the fraud, the costs and how to target some of the big areas of cost, and reduce those. But with AI, they’ve taken it to the next level, and their latest model is probably saving them somewhere around, sort of £5 million a year on fraudulent insurance claims. And they’re using AI to just really look for patterns in the details of the claim and spot those patterns recurring over time. And the next step is to start looking at the voice and actually not just what the notes are that go with the claim, but actually what the customer is saying and analysing at that level.
David Naylor: So you’re going right back to the source rather than just the written content of a claim. So that’s getting possible now because the speech analytics capabilities are improving so much. And you see other examples of phone printing technology, which is supporting organizations to actually not just listen to the voice, but listen to the stress and listen to the way in which customers are speaking and how they pause and respond to the questions. And combine that with lots of geographic data that sort of sits behind the call. So, what number are they calling from? Does that number match up with our data? And things like that. So you can start to get a much bigger picture of potentially fraudulent claims by pulling all those discrete bits of data together along with the more AI-generated bits of data and start to look at the pictures and patterns in those calls. So it’s definitely a really big growth area, and a number of key vendors are offering that sort of technology today already.
Charlie: Yeah, I think I remember speaking to one person and they said that this AI technology can pick up hundreds of characteristics just in somebody’s voice alone and then match that with other patterns of the customer’s speech and activity. As you said, it seems like a very useful thing and definitely probably the future in fraud protection. And another area where I see AI coming in a lot at the moment is in routing, and predictive routing especially. Can you just tell us a little bit about how AI can be used for doing that?
David: Yeah, I suppose it’s building on the modelling that we talked about earlier, but putting it into a live interaction environment where you’re bringing in data from the historical context for a customer and looking at the interactions they’ve had with you. Have they been on the website? Have they been looking at particular products? And all that sort of data that we want to try and bring together into one place. There’s certainly progress being made in being able to capture that and make use of it. I think the other side of it is when the customer calls in, is asking them what they actually want. We make assumptions about what they want based on whether they’re in a renewal cycle on an insurance policy, or we can see that they’ve been on the website. But actually then combining that with the audio and saying, “so, how can we help you today?”
There’s lots of advances in that technology space which are really interesting today, with open platforms providing the speech recognition and intent analysis which allow people to get started. So there’s a really easy, as I mentioned, thing for the likes of Google and Amazon, IBM, others are all providing these solutions which get very… which help you to get started, I suppose. I think there’s still an important point around professional services and making sure that these solutions are robust. But you can certainly experiment very easily with creating your own customer interaction solutions. So yeah, they’re great because when we ask the customer what they want, we can record that snippet of conversation and instantly you’ve got some categorization of call types. So I want to talk about… I want to cancel my policy, I want to change my vehicle, would be the sorts of things that customers would say in the insurance world.
David: And then from there, you can use some self-service technology, which allows you to fulfil that need without actually needing to speak to a customer service representative. And the capability with AI-powered speech engines today means that the accuracy and effectiveness of those solutions is very good. So just for one client, on case study of a client who has implemented this, just a routing solution to help them get the calls to the right place, whether it’s going to a store or whether it’s going to a contact centre, big retail client. We’re routing 11 million calls through this technology and have dropped their transfer rates down to really, really low levels. I can’t share the numbers, but they’ve done an amazing job at reducing call transfers because of misdirected calls. So there’s certainly a real business case to be looked at for this type of technology, whether it’s just for simple routing or whether it’s for full self-service.
Charlie: I think it’s also a very good example of how AI is not just about customer data and how you can use advisor data as well. And then route the customers to advisors who they’re most likely to have great rapport with. So it’s definitely a promising area. But one that our listeners might be a little bit more familiar with is chatbots, of course. But one of the key trends I’ve noticed with chatbots recently is that they’re being used more for agent assist as opposed to being a channel in their own right. Why do you think this might be?
David: These solutions need training and they’re not just things which you switch on and say, all right, listen to what the customer says and respond here, which is what Microsoft did. Go and learn about the world, effectively was the goal. And within 24 hours, this chatbot was taught to be racist and all sorts of other things, which as you can, you can go and search the Tay chatbot online if you want to find out more about what happened. But it just illustrates the point that actually sitting behind a chatbot is a lot of work to build conversations that actually work for a customer. You know, there’s no point creating a chatbot which, in nine times out of 10, they’re not solving the customer’s problem. You’re not containing the conversation, if you like, because you’ve then got the cost of the chatbot and then you’ve got the cost of the follow-on contact to fix for what the chatbot didn’t resolve.
So conversation design is a critical part of that. At the moment, there are too many bad chatbots out there, customer-facing chatbots. So the benefit of using agent assistance first is that you can effectively use the agents as your safe test ground, if you like, for developing a chatbot, which just sits and listens in the background, or watches in the background, for the conversation that the agent’s having with the customer. And then make suggestions. So it can be quite supportive and can supercharge the agent’s capabilities. But this certainly speeds up the process by suggesting things that the customer… that the agent may need to help solve the customer’s problem. So if the customer is saying, I’ve got a question about my bill, I don’t think the rates on my tariff are quite right, for example for a water company or electricity. Then as it hears tariffs, it can pop up the link that says, okay, here’s the link to the tariffs that are relevant for this customer.
David: So, that might not be the thing that you need at that point. But hopefully as time goes on, it will learn more about what you do need and support the agent to resolve that interaction quicker. And so once you’ve done that in the safe environment of an agent, you can then take that learning, and it doesn’t necessarily translate directly straight into the outside world, but you learn a lot about how to develop an understanding of language and interaction. And from there you can then build the self-set applications that effectively represent how the agent works rather than what the process should be. Because at the end of the day, it’s how your agent is interacting with customers is actually the important thing, rather than trying to stick to a script which doesn’t necessarily work in the real world. So that’s the benefits, in my head, of using the agent to support this process of learning and developing before you get to putting chatbots out into the customer world.
Charlie: Yeah, I think it’s interesting from what you said, it seems the contact centres are becoming more savvy as to the misconceptions that were there a few years ago. Are there any other chatbot misconceptions that you’ve come across? Maybe, such as that chatbots can detect sarcasm? Are there any other kind of myths out there that you believe are still quite commonplace?
David: I mean, I think the key myth is that these things are self-learning. So you know, there’s quite a lot of clients who have been, have seen demos of systems that they can watch what you do and then copy what you do and improve. And there are certain elements of that which are true, but what they are doing is they’re collecting that information and then it’s going back to a human, to a trainer, if you like, to say yes, what you’ve learned here is correct. So add that to your process. No business would be automatically allowing a chatbot to update itself. So it’s got to… you’ve got to go through that human-in-the-loop process, whatever happens. So automated sort of services, in that sense and not that automated.
Sentiment, sarcasm, again, it’s down to the capabilities of the language model that sits behind your chatbot, and again it’s detecting some of those really contextual things which we take for granted as humans, and we just know when someone’s being sarcastic. The systems can’t do that. There’s no system that in a production-level environment can detect sarcasm that I’ve ever come across so far. That’s the next level of conversational development that we will get to with development of language models. And there’s lots of research going on about how to improve those language models. But it’s in the lab. It’s not in the real world at this point in time in any robust way. The final thing is just about how much AI actually sits inside these models. So the AI sits in the language side, so understanding what the customer’s asked for. From there, you’re kicking off effectively a script. So you’re running through a process. So if I want to do an adjustment on my car insurance, the AI is detecting the language that you’re using.
David: So, I want to change my vehicle. And from there, we go into a process that says, right, these are steps we need to go through. You do it well, you can make it a good conversation as opposed to a “now give me this information, I give you that information”. But that requires a lot of design work from people. It’s not something that machines will do by themselves. And you wouldn’t want a machine to do it because it wouldn’t work out well. There are tools to assist. There are tools which will take lots of examples of chats, for example, the live chats we’ve cut with agents, that represent that process. And they can make suggestions about how to design this process well. I have to design this process based on what they see, but ultimately there’s a lot of work needed from the operation to make these things effective.
Charlie: Yeah, I think particularly actually, moving on to some success stories now of chatbots that have been implemented. One that I’ve personally been quite impressed by is Lidl’s Margo, but that only works in one defined scenario of recommending food and wine pairings. Do you think at the moment that chatbots working for one defined scenario is the ideal strategy for an organization?
David: It’s the only strategy, I’m afraid. In the sense that you’re training something to do a certain task here. So once you’ve kicked off a process that says, right, this is about car insurance adjustment or a change of address. Within this car insurance sector, then you can build these scenarios in the chatbot and the language model is tuned for that industry, for those products, et cetera. But you wouldn’t on the same platform be able to put your wine bot, for want of a better phrase.
That’s very much a separate application and so it’s really down to some very narrow forms of AI at this point in time. And yeah, there are some really good examples, right? And I think people like booking.com have done a really good job with the support of their chat around the process of post-booking, pre-visit, and done a lot of work to make that chat support there do a job, a really specific part of their process. Yeah, it’s not even covering… so the end-to-end process that I’m looking for somewhere to go, or post-interaction process to post-visit process. It’s all about ensuring that issues that you have in that stage of the process of your experience are dealt with very well. So you have to really think about where you’re going to get most bang for your buck in terms of developing some of these applications. And the more focus, the better at this stage, I think is definitely a key thing.
Charlie: It’s interesting how the chatbots are being implemented at the moment. And a lot of it is to take up the transactional queries, that contact centre space, the simple transactional questions that they get posed. Do you think over time this will start to have an impact on the job of the call centre advisor?
David: It certainly will. And I think we have to accept that automation in the contact centre space over many years and that that has continually taken away some of the lower-complexity tasks. And we’re seeing things like robotic process automation combined with AI, which I guess is quite a different thing, working to reduce some of those tasks as well. So I know of one particular client in that space who’s deployed some RPA into their operation to bring together all the different systems and make it simple for agents to access, to kick off processes, to reduce the effort in that process. They’ve reduced the headcount needs by 25%. That’s a great success in the sense of cost, but clearly it has an impact on the contact centre. There are not many cases where we’re seeing that sudden step change in need of resourcing.
David: I think we’re going to see a very gradual impact of AI. And I think, personally, I think the majority of that impact will be handled through natural attrition in the contact centre rather than sudden job losses for most clients. So what that then means for the resources that’s still there, which is a good proportion of people really, is that the tasks they will be handling will become more complex, and therefore the power of the agent-assistance tools really comes to the fore then, because you’re dealing with the more complex issues, and anything that you can do to help to make that easier for the agent is clearly a good thing for the customer as well. So there’s lots of bits coming together that AI is helping with across that whole experience, whether it’s taking some tasks and fully automating them or it’s just supporting the agent.
So the wider question of what does that mean for us as a society and, you know, the numbers of jobs that AI will impact and take away, I think no one’s got a real answer for that at this point in time except that, in my view, we will be creating a lot of new jobs to support the development of this technology, and it will open up new jobs as technology always has done. So I’m a real supporter, as you can imagine, of what the technology can do, and I hope, and I believe, that it will be supporting society for good, not just taking away jobs today.
Charlie: Yeah, it’s interesting. I think contact centres have a lot to think about maybe in terms of lowering occupancy, so advisors get a longer time between calls if they’re longer calls with greater complexities. So there’s definitely lots to think about.
And I think a key topic that we haven’t actually discussed yet, that you mentioned there, was robotic process automation. Do you think it’s good to have some sort of RPA in place before an AI solution is deployed?
David: I think it’s good to think about them both together, actually. RPA is very dumb in many ways, but it’s simply helping you to take away key presses on a desktop for an advisor. What it can’t do is make any decisions except some basic logic decisions of, have I collected all the data I need to collect from different systems, and does that data make sense to pass onto the next stage of the process? If there’s a decision to be made at that point, which is are we going to approve something or are we going to confirm that a customer can shift across from one tariff to another or something like that, then there’s some decision logic needed.
David: Now maybe that decision logic is quite simple, and it’s just based on meeting a few criteria, or it could be more complex. And normally if you have those complex ones that require a human to intervene and make a decision, obviously the RPA just kicks those out into a queue, and someone will go through them and decide on, yes, no, yes, no. So if you can’t automate that, because it’s quite complex, then clearly there’s still quite a bit of work that needs to be done manually. But over time, I think if you sat down and went through those decisions and said, right, actually we could probably build some AI that could give us initially maybe 80% certainty in 20% of cases that the decision that we’re going to make through AI is correct. Then let’s build a model that does that because it then takes out 20% of those bits of work that are queued to the human for decision-making.
And over time you can improve that model and it will give an 80% certainty 30% of the time, et cetera, et cetera. So for me, I see that RPA has some glue in between the different bits of it, which is provided by AI, and the human. And over time the AI will be able to do more of those decision-making steps and allow the human to focus on the really complex stuff. So I think putting them in together is a way to think about it. That sounds significant in terms of effort and it is, but there are clearly, I think for me, RPA can be done… the bit that’s just automating the current process can be done by itself. You then get the extra benefit by putting the AI in between the two. And I think the organizations that provide RPA today are starting to think about that, but they’re not there yet. They’re more focused on… there’s still a massive market just for automating some of the process. And in time, that next step will come, but I think they will start sort of flowing together and delivering significant benefits in unison, really.
Charlie: Yeah, I think that certainly matches what I’ve seen in terms of automation being introduced at the moment. It is very much in the back office and yet to be brought really to the forefront of contact centres. So it would be… hopefully we will see that happen. But in terms of AI being deployed on a wider scale, which sectors do you think will be the first to introduce AI solutions en masse, if you like?
David: Yeah, so everyone talks about customer service. When you see charts that show where the benefits… I mean the customer service does always come up near the top of those charts of potential benefits. I think there’s some areas where we’re starting to see more real-world examples of delivering some significant benefits, like in the healthcare space. That’s very much a sector that is using both image technology supported by AI as well as decision-making to supercharge the process of diagnosis, for example. Again, you got to be very careful with that and make sure that if there’s any doubt then it’s escalated. But people like Babylon Health, who are a company that you see a lot of based in the UK, doing a lot of work with the NHS to develop solutions and services in the healthcare space. So that’s worth looking at.
David: And I think in financial services specifically, I think the area is around fraud, and managing that aspect of risk is a big area at the moment, and you know that because there are clear financial benefits right off the bat if you can save 10% of your losses in fraud, their business case is very clear. And so that’s getting some really good traction. I think with customer service, we’re in that game of how do we introduce channels cost-effectively, or chatbots or a new channel, without creating more work. I think people introduced webchat, they were supposed to reduce contact and provide a lower cost. I’d say that most people still see webchat as a channel which is the same cost as a phone channel. And you’ve got to be very… it’s quite challenging to prove that you’ve reduced contact as a result of a webchat because you’ve got to link data together.
So I think some of the elements of proving the value in contact centres still has some way to go as opposed to these other areas where we’re seeing it progress quite fast. The applications across industry – this is not a technology that sits in one space – it’s obviously, we see it in the automotive space, in self-driving cars and those sorts of things, but we are a long way from having a self-driving car on the road at this point in time. And I think there is some… I’m not saying we’re at a false dawn – that we’ve been through what was termed an AI winter between, I guess, when I did my PhD and 2010 perhaps. And there’s a danger of it being oversold in some areas. And I think what I am trying to do, and others, is to try and provide, produce some realistic benefits for clients and help set realistic expectations and show those benefits in real terms, in terms of savings or customer experience improvements, which are really important.
Charlie: Excellent. Well, I think there’s a lot in there for us to take away. I certainly learned a lot today, so thanks, David. Where can our listeners find you if they wish to find out more about implementing AI in their own contact centre?
David: So, if you look at the website, which is humanotics.co.uk, or drop me an email, david.naylor@humanotics.co.uk, happy to have a chat and just answer any questions, even if it’s just tell me a bit more about AI. I want to understand what this means or that means. Always good to have interactions with people about that. So yeah, those are the ways.
Author: Jonty Pearce
Published On: 19th Aug 2019 - Last modified: 22nd Apr 2024
Read more about - Podcasts, Artificial Intelligence, Charlie Mitchell, Genesys